An economy that puts back what it takes out, that is sustainable by design. — Matthew Bishop
The circular economy is a foundational principal of sustainable commercial and industrial activity.
Circular economy requires that we account for the natural resources we take and use in everyday processes and, as far as possible, return them to their original state — everything from air and water to habitats. Further, every product — every single product — we consume needs to have a reuse or recycling pathway either directly or in an adjacent process or industry.
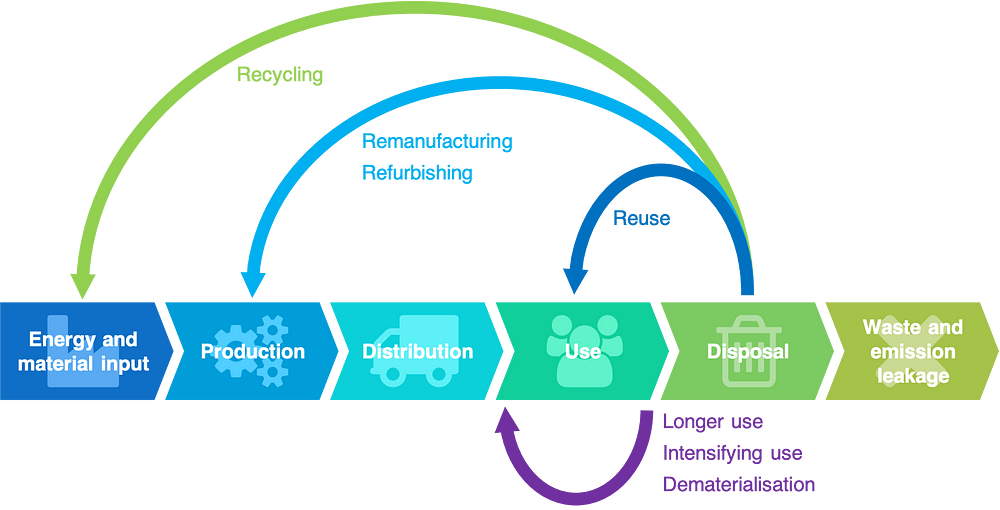
Be in no doubt, the transformation required by the circular economy will make the industrial revolution and the data revolution look like a walk in the park by comparison!
What does circularity entail?
In its industrial processes, a truly circular economy creates no net pollution or waste. — Matthew Bishop
Given this stringent definition, the goal of achieving circular industrial processes across a national and by extension, for it to make sense, a trans-national economy, is a huge challenge. A social challenge certainly, a commercial and investment challenge definitely, but also I argue, a data challenge and and a massive scientific challenge as well.
Circularity subsumes the carbon cycle neutrality that many nations are working towards and extends it towards other natural resource cycles such as water, air, minerals, and biodiversity. By definition though, circularity includes the products that are created in a thriving economy and all the materials that go into making them.
If circularity is to happen — and for ongoing sustainability it surely has to — this requires the a dramatic and disruptive change to the global economy.
Changing production/logistic networks
Currently many products and processes revolve around a linear production model. This does not work well as a model for reclaiming materials for recycling and reuse as many products end up in landfill.
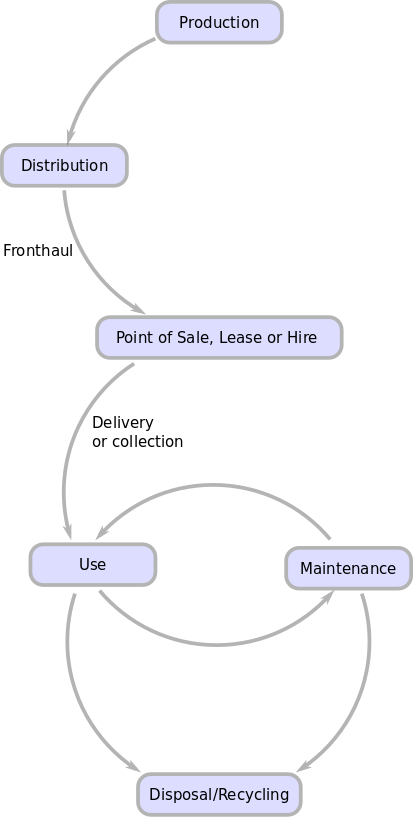
A linear production model involves a supply chain of raw materials that see a product created at a production facility and then pushed into a supply chain distribution network. The use-life will sometimes have maintenance cycles embedded in it if the product is of sufficiently high value. When end-of-life is reached the product is disposed to landfill or incineration with some portion of the product being recycled depending on legislation, consumer habit and process availability.
For new products designed for circularity, we will see many different and potentially disruptive production and use models spring up.
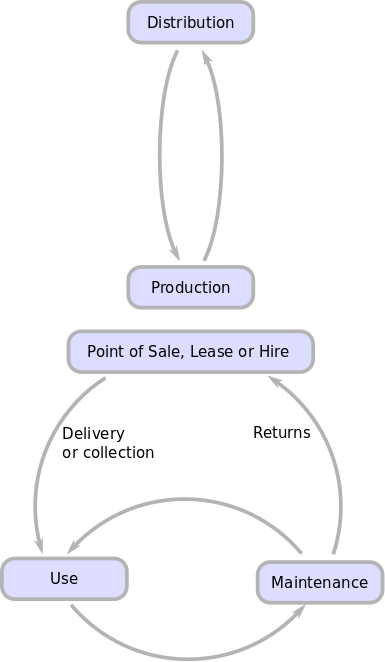
Future circular logistic chains may see production — perhaps micro-production — move closer to the point-of-sale. Imagine bottling plants or food packing facilities embedded in supermarkets.
Similarly some outlets will become points-of-return for their products at end-of-life. Except that, what was previously end-of-life will become start-of-second-life after a suitable recycling process.
The distribution network will become a means for front-hauling raw components to the manufacturing facility and taking packaging and old products for refurbishment — so-called, back-haul.
All this requires unprecedented cooperation between logistics providers, manufacturers retailers and consumers. Undoubtedly incentive programs like bottle deposit schemes will be needed and the economics of even those industry schemes are not straightforward. For more expensive products, lease-and-return will likely be the better model replacing own-and-discard.
The scientific and engineering challenge
Let us for a moment look at the industries that will be swept up in this revolution:
- Building and construction
- Clothing and textiles
- Metals (bulk metals like, iron and aluminium) as well as tech metals such as rare earth metals, copper and lithium
- Farming and food production
- Energy generation
- Water processing
This is a scientific challenge at a materials level, and an engineering level at a process design level. Assuming we have a circular supply chain in place (see above) to collect the raw material: Can we physically or chemically reconstitute the materials once they have been used? Does an industrial scale process exist to support such a reclamation? Is it economically viable to carry out the recycling activity?

As an instance, thermal recycling of glass (melting recycled glass down and reforming it) cannot be done currently with 100% used feedstock or cullet (the name given in the industry to the broken glass obtained from bottle banks). It has to be mixed with new silica/soda-lime in order to produce a suitably high quality product (green glass can be formed from 90% feedstock, clear glass only 25%). Different but equally hard challenges exist in recycling PET plastics and other raw materials.
These are massive challenges by themselves and the good news that many research funders and universities are making inroads into these challenges.
Why a data challenge?
If this wasn’t exciting enough, the cross industry aspect is where the circular economy becomes a really interesting puzzle.
There is no such thing as ‘away’. When we throw anything away it must go somewhere. — Annie Leonard
The challenge lies in joining up industries that have previously had no interrelationship.
For the sake of argument, say it were possible (I’ve no idea whether it is) to reuse the magnets in the motors of discarded washing machines and tumble dryers to help create generators for wind turbines and new motors for electric cars. This would be an example of how the white goods industry might find itself in the supply chain for fast growing green technologies.
If we now forbid those growing industries to use virgin raw materials from usually mined ores — we run into two problems straight away:
- Are there enough discarded washing machines and tumble dryers to supply all the magnets need by the wind turbine and electric car industries
- Are the recycled materials available in sufficient quantity at the right time to allow manufacturers to rely on them in their supply chains
Even if the answer to (1) is yes, the answer to (2) is almost certainly going to be no — at least to start with. These industries have never had cause to synchronise before — they are not aware of each others production flows, but they will need to be if there is to be synergy between them. This is where data science and smart supply chain processes can drive real innovation.
Data flows for circular economy
In supply chains, products, components and raw materials flow between mines, factories, warehouses and points of sale. In efficient supply chains, goods do not spend long at any one point in the supply chain, instead they are ordered as needed and in anticipation of a downstream demand.
What drives this efficient flow is data pipelining and accurate forecasting of demand. Without that, materials end up being stock piled in warehouses to mitigate the risk of material exhaustion. Storage of materials, just as with components, is inefficient and costly.
In a circular economy with many cross-industry dependencies, we will need data visibility on:
- demand forecasts in consuming industries; and
- flows of products reaching end-of-first-life in feeder industries
- volumes of recycled material that is about to be available for reuse
Industries which have never talked to each other will need standardised APIs for interrogating each other or pushing notifications to each other to achieve this seamless integration.
Algorithms for the circular economy
If this sounds like it will be ripe for application of predictive data science, it absolutely will be. The better the forecast or anticipation of arrival of a feedstock, down to the day, hour or even minute, the more efficient the manufacture of the new product can be. Materials arriving early can at least be stored (if space allows) — materials arriving late though will potentially hold up a whole manufacturing pipeline.
Another key technology will be in the area of meta-heuristics, e.g. Silva et al. 2003. If you’ve never heard of these before in the context of data science problems, they are approximate but fast solution approaches to massive multi-scale optimisation problems. And if we are to minimise the wait time, maximise the throughput and minimise the waste in dynamic cross-industry supply chains, we will need the very latest state-of-the-art optimisation algorithms to enable this.
Models of flow of goods than can capture recycling streams at a cross industry level will be extremely important, for example, Bhaskar et al. 2010. These models will need to represent delay, storage and uncertainty but they will also need to learn distributional patterns that represent volume. Being able to identify key bottlenecks well ahead of them actually occurring will be necessary so that alternative sources or feedstocks can be planned for.
Conclusion
So what can we do now to address these challenges? For those of us who look for data-driven projects in a variety of industries — there is low-hanging fruit and much of the benefit can start within a company.
- understanding and mapping the data flows and data services within a company is a health check that many companies could do and leave for another day
- creating a sustainability call-to-action within a company which looks at the flows of natural resources as well as raw products and would allow for an audit of natural capital expenditure will likely expose circularity shortfalls
- engaging with the data science to create high quality forecasts for many parts of the company to use and understanding the key impacts and risks of that forecast being right or wrong
- engaging with universities and circular economy hubs at this early stage so that a company is aware of upcoming technical and business opportunities
- creating an open data culture so that people who want to work with you can see your consumption and your production and forecasts of the same so they can understand what the time and volume challenge will be if they start to be your supplier/consumer for a critical material
If sustainability is to be sustainable then it has to drive value itself and the good news is that the actions that any company can take in preparation for the circular revolution will also drive efficiency, savings, internal reliability and external opportunities.
References
Geissdoerfer, M., Pieroni, M.P., Pigosso, D.C. and Soufani, K. “Circular business models: A review.” Journal of Cleaner Production, 2020.
Silva, C.A., Runkler, T.A., Sousa, J.M., Sá da Costa, J.M. “Optimization of Logistic Processes in Supply-Chains Using Meta-heuristics.” EPIA 2003: Progress in Artificial Intelligence. LNCS vol. 2902, pp 9–23. Dec. 2003.
Bhaskar, V., Lallement, P. “Modeling a supply chain using a network of queues.” Applied Mathematical Modelling 34(8):2074–2088, Aug. 2010.
DOI: 10.1016/j.apm.2009.10.019
About the author
Dr. Jeremy Bradley is Chief Data Scientist to Datasparq, a member of the Strategic Advisory Network for the UKRI/EPSRC Circular Economy Theme, a member of the Strategic Advisory Team for EPSRC Mathematical Sciences and Fellow of the Institute of Mathematics and its Applications. He lectured and researched Computer Science at Imperial College London for 14 years. He has industrial data science experience as part of the data science teams at Tesco, Royal Mail and now DataSparQ. Jeremy also co-hosts the DataCafé data science podcast with his friend Dr. Jason Byrne.
If you would like to know more about how sustainable data science can help your business then do get in touch. We would be happy to share our learnings and see if we can help.